Welcome to the the web supplement for the manuscript:
Proteome-scale detection of small-molecule protein interactions using data from gene expression perturbations
Using the left navigation, you can view supplemental information. The code and binary are also available.
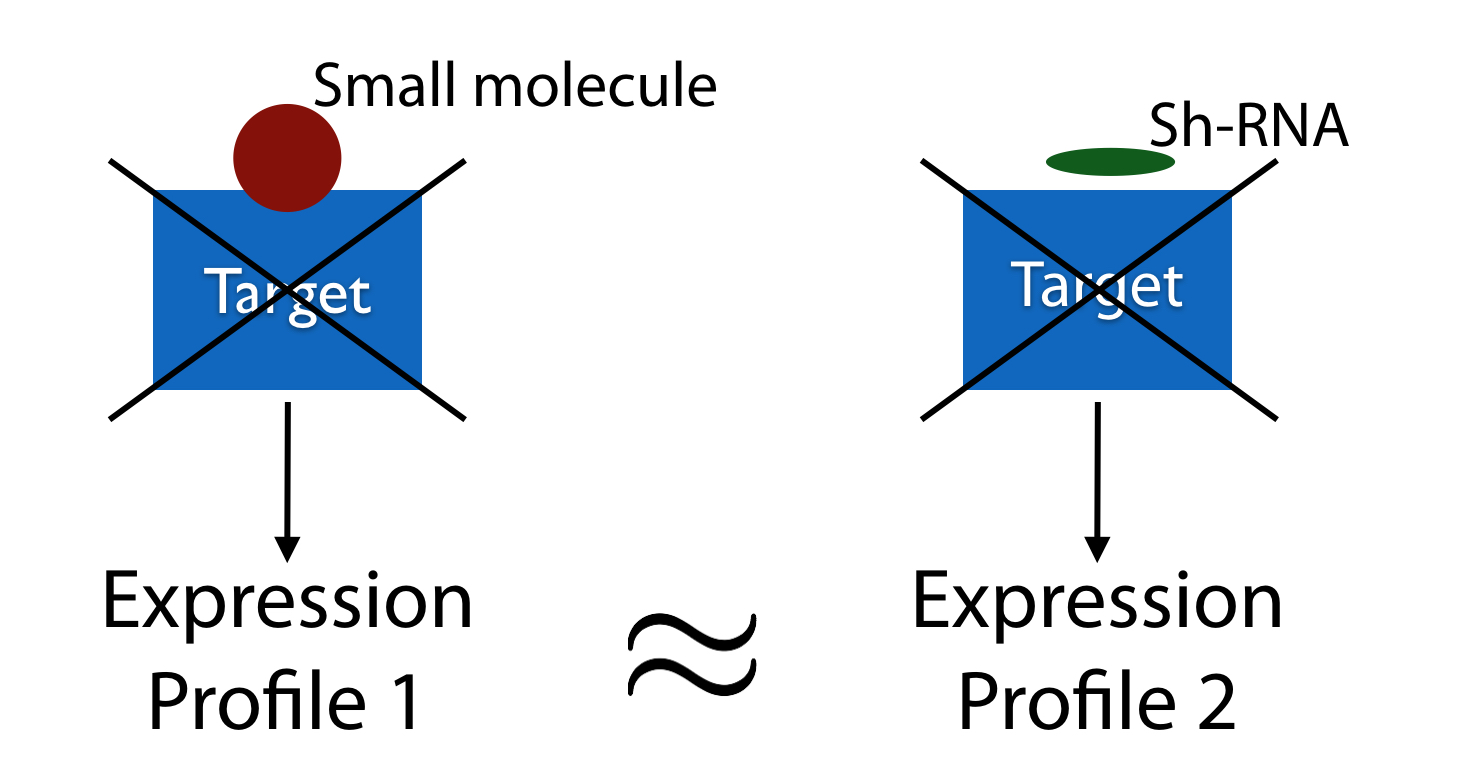
ABSTRACT
Delineating the roles of small-molecules in perturbing gene expression in normal and disease states represents an effective route to identify new targets and chemical strategies for drug development. Here, we present a high-throughput virtual screen to identify drug targets based on cellular gene expression profiles from millions of knockdown and drug treatment experiments. We train a random forest classifier to recognize drug-target interactions encoded in correlations between these profiles, and refine these predictions using an orthogonal structure-based screen. Cross-validation on a set of 152 FDA-approved drugs and 3104 potential targets identified the known target in the top 10/100 predictions for 26/41% of the drugs. We apply our method to 1680 small molecules profiled in the NIH LINCS library and experimentally validate novel targets for five drugs. Our pipeline has the potential to accelerate drug discovery by evaluating previously uncharacterized compounds that simultaneously inform on multi-target effects, toxicity and disease phenotypes.